In this paper we explore how quantum scientific machine learning can be used to tackle the challenge of weather modeling. Using parametrized quantum circuits as machine learning models, we consider two paradigms: supervised learning from weather data and physics-informed solving of the underlying equations of atmospheric dynamics. In the first case, we demonstrate how a quantum model can be trained to accurately reproduce real-world global stream function dynamics at a resolution of 4∘. We detail a number of problem-specific classical and quantum architecture choices used to achieve this result. Subsequently, we introduce the barotropic vorticity equation (BVE) as our model of the atmosphere, which is a third-order partial differential equation (PDE) in its stream function formulation. Using the differentiable quantum circuits algorithm, we successfully solve the BVE under appropriate boundary conditions and use the trained model to predict unseen future dynamics to high accuracy given an artificial initial weather state. While challenges remain, our results mark an advancement in terms of the complexity of PDEs solved with quantum scientific machine learning.
Potential of quantum scientific machine learning applied to weather modeling
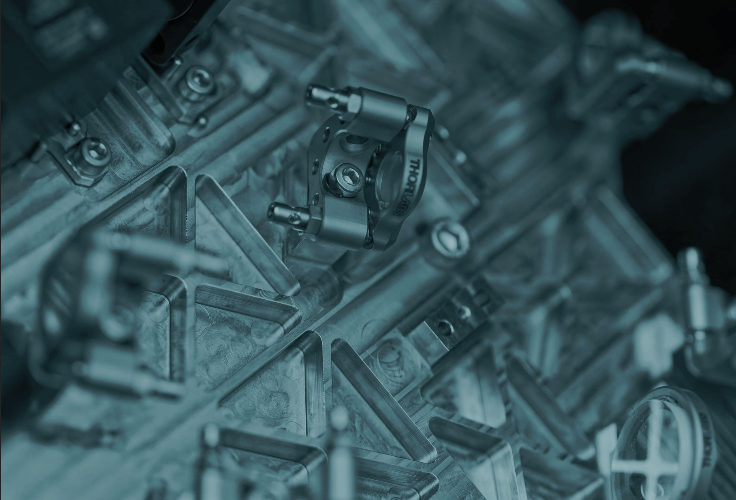